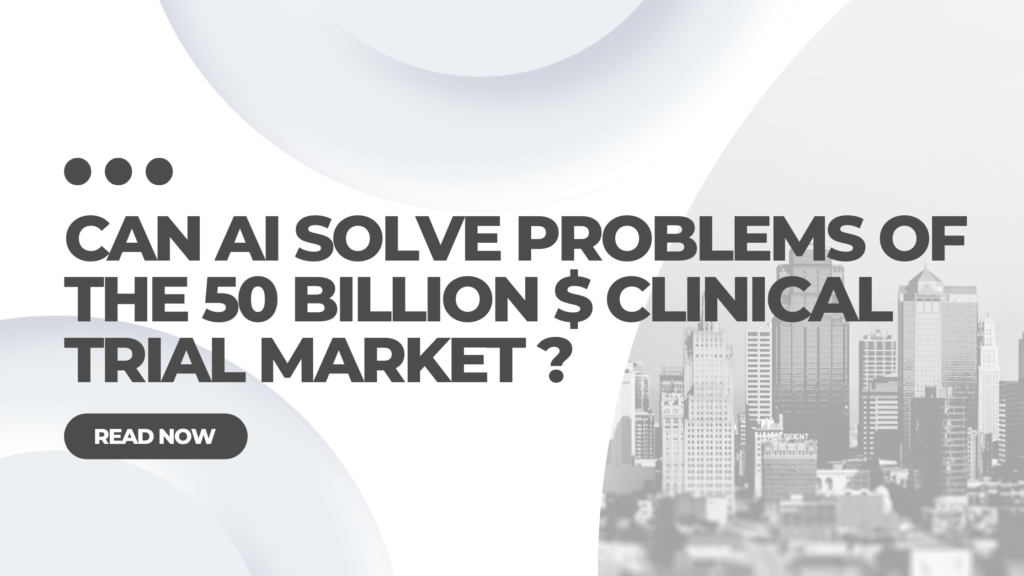
The power of AI in clinical trials: the COVID scenario
“It saved us an entire month,” says Demetris Zambas, Vice President and Head of Data Monitoring and Management at Pfizer. “It really has had a significant impact on the first-pass quality of our clinical data and the speed through which we can move things along and make decisions.”
This was just for one miniature step of COVID vaccine development that saved several precious lives from being lost and largely reduced the burden on the healthcare system and workers. Scientists at Pfizer used a machine learning (ML) tool called Smart Data Query (SDQ) to reduce the at least 30-day process of database lock to just 22 hours. Artificial Intelligence (AI) and ML tools have helped COVID response not just via better data management but also by quicker drug discovery, identifying key locations to set up trial sites, identify patient characteristics that are more likely to respond to a specific treatment pattern and several others.
Clinical Trials Market Projections
Given that we have a stark example of how AI can help several parameters of clinical trials, it is important to put this into $$ figures for which we need to firstly know how big the clinical trial market really is.
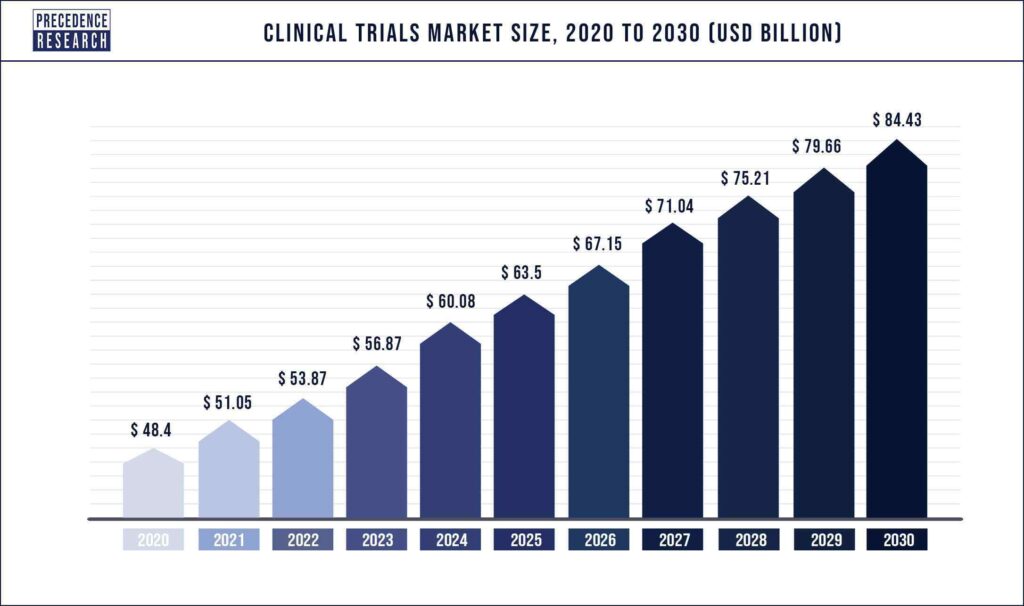
The current market size is approximately 50 billion $ and is expected to reach 85 billion $ by 2030. North America is the fastest growing market due to increased research and development investment and government assistance. Phase III trials and oncology trials dominate the space and this trend is expected to continue. The increase in market is also largely influenced by the availability of increasingly advanced AI and ML predictive models.
What is the time and cost of the clinical trial?
On an average, to bring a new drug to market, it takes 10–15 years and 1.5–2.0 billion $. Of the total, about 50% of both time and investment is spent in the drug development cycle. The remaining expenditure covers preclinical compound discovery and testing and regulatory processes. This timeline is due to the traditional flow of process and data across the clinical-trial life cycle, which can be a combination of manual effort, rework, miscommunication, and inefficiency.
Only 10% of the compounds that enter the clinical trial stage advance to regulatory approval. The high failure rates of clinical trials contribute substantially to the expenditure. This trend in failure has been observed for decades and is ongoing.
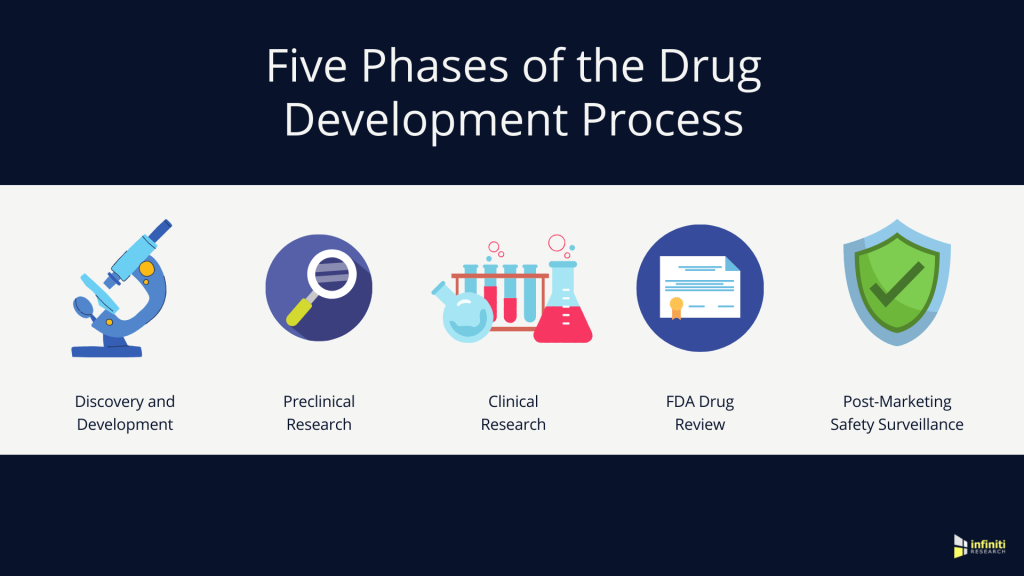
What are the challenges of clinical trials in the current times and how AI can solve it?
There are several challenges with the clinical trial space that an AI model could solve. Here, we will focus on three major challenges that underpin the wastage of time and money in this market.
Challenge No. 1: Cohort composition
One of the most common issues with every single clinical trial is selecting the right patient. An ineligible patient is defined as those who are not in a specific group, stage, or the phenotype of the disease. Even when a patient becomes eligible, several road blocks including lack of proper incentives, unaware of the matching trial and recruitment process, or complex recruitment process prevent the early and adequate patient selection delaying completion and failure of up to 32% of the trial. Untimely patient selection and recruitment into larger randomized clinical trials have serious consequences leading to increased resource use and costs with the length of the trial being extended.
Clinical Trial Enrichment is a strategy used to specifically select a subset of the population that can more readily demonstrate the effect of a tested drug. If this enrichment is not made, the observed efficacy of the tested drug will automatically decrease. Although clinical trial enrichment is not mandatory in patient selection, failure to use can certainly increase the likelihood of failure rate.
AI solution:
AI models that can combine information from scattered electronic medical records (wearable medical devices, hospitals, local clinics) and genomic data will help identify the therapeutic targets for specific drugs. This can lead to measurement of specific biomarkers and selection of patients based on biomarker values. AI models, in general, are data source-agnostic; this characteristic could be valuable when harmonizing highly variable electronic medical record data from different sources. Preclinical compound discovery and patient cohort selection can be improved using AI models via search for effective correlation between indications and biomarkers and reduction of patient heterogeneity, prognositic enrichment, and predictive enrichment, respectively.
Challenge No. 2: Patient recruitment and enrolment
Trial eligibility criteria are complex in terms of number and medical jargon making it challenging for a patient to comprehend and assess their own eligibility. The process of traveling to trial sites can be burdensome and time consuming for trial participants, which can negatively affect enrolment.
AI solution:
Natural language processing (NLP) is a computer science model that combines computational linguistics with statistical, ML and deep learning models. NLP enables computers to ‘understand’ the full meaning of human language ( text or voice form). AI models that implement NLP can help simplify clinical jargons and make it more digestible for both the site personnel and the patients. There are currently several digital health companies that use AI models to find rare disease patients (like needle in a haystack) for clinical trial participation. AI-powered wearable devices can lessen the need for participants to travel to a physical site, which can enable organizations to recruit patients and diversify clinical trials participation.
Challenge No. 3: Patient monitoring, adherence, and retention
Recruited patients need to complete dosing and follow-up to have meaningful data analyses at the end of the trial. Patient attrition during the monitoring and follow-up period is inevitable with only 15% of clinical trial not experiencing a dropout. A study where half of the subjects drop out will need twice the amount of patients to be enrolled. Frequent visits to trial sites may become invasive and unpleasant, leading participants to drop out of trials.
AI solution:
Wearable sensory devices and audio and video monitoring models have already provided enormous data over a period of time to monitor clinical variables in subjects. These devices can further be modified to specific clinical trial needs relieving the patient of the task of collecting their own data and keeping track of it over different timepoints. Deep learning models can be used to analyze such data in real-time for detecting and logging events of relevance as well as a stored data to inform future clinical trials.
In addition to solving problem related to patients, AI can largely reduce the time it takes to gather, clean, and analyze the giant amount of data that is collected.
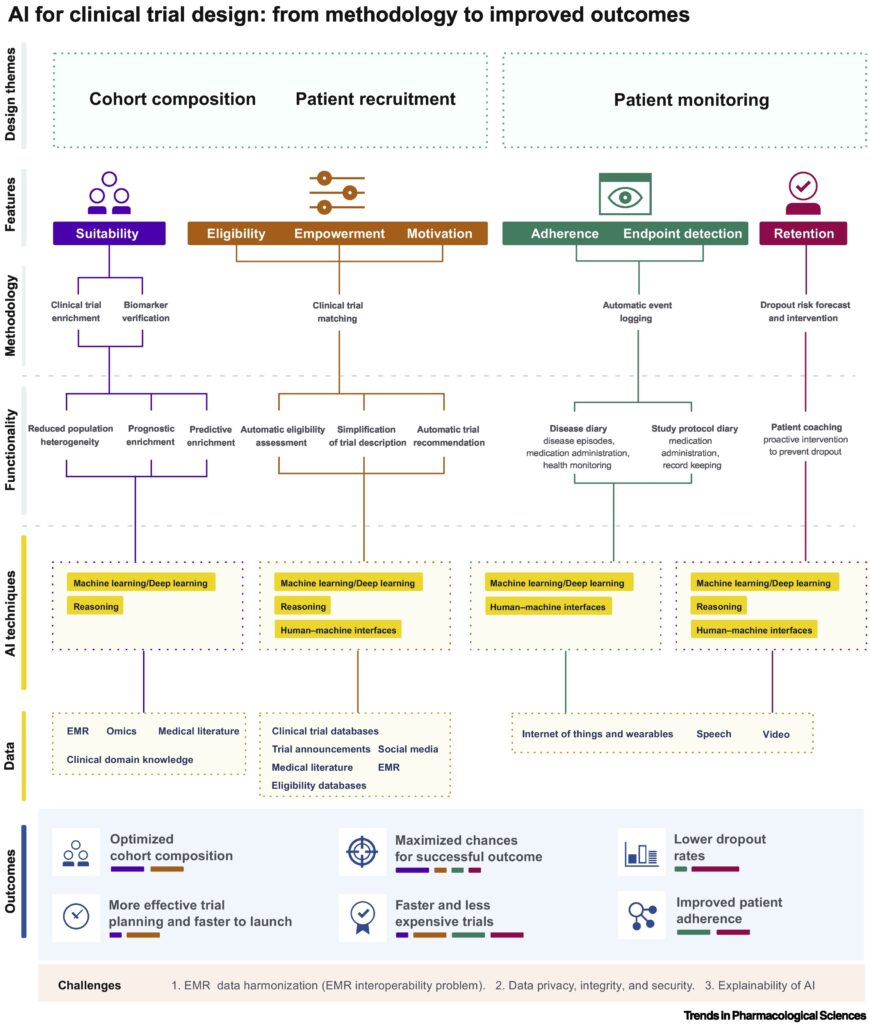
Ideas for potential AI solutions
- Collation and management of available data
A model that can mine large data sets available across institutions, across countries, across various electronic health systems and give insights into appropriate patient selection, enrolment, and visit management will add significant value to the clinical trial ecosystem.
- 2. Encryption of patient identity
Safeguarding patient identity can facilitate sharing of data between researchers enabling collaboration and development of better AI models.
While there are still several challenges in developing and implementing successful AI models in the clinical trial ecosystem, the promise of AI in its ability to analyze large datasets that is otherwise humanly impossible gives us hope to see shorter and less expensive clinical trials at least within the next decade.
Reference
Trends in Pharmacological Sciences, August 2019, Vol. 40, No. 8